Payment fraud: How machine learning can help protect your company
Around 47% of companies have been a victim of payment fraud in the past two years (2020 Global Economic Crime and Fraud Survey, 2020, PwC). Unsurprisingly, 49% of companies are more concerned about payment security now than they were in the year prior (2020 B2B Payments Survey Reports, Strategic Treasurer).
Fraud prevention is a company-wide effort. An effective anti-fraud strategy consists of three core elements: security policy, people, and technology. Security policies have to be clear, comprehensive, and well-defined plans, rules, and practices to protect a company’s information and systems. These days, employees need to understand security measures and be able to identify cyber threats and attack scenarios. Technology can also prevent certain types of fraud and can help stop and prevent others.
Treasury technology to increase data security
When arming up against cyberattacks, using a Treasury Management System (TMS) is a good starting point. These tools automate bank connectivity and make global cash flows visible in real time. They also include many features to increase data security. Here are three examples:
- To prevent cybercrime and internal fraud, you can define user rights and roles, payment signatories, approval workflows, and payment limits in a TMS.
- To increase data security, treasury software uses data encryption, two-factor-authentication, audit trails, and password controls. Allowlists and denylists are used to increase payment security.
- To identify data breaches in real time, sophisticated TMS use machine learning.
Machine learning techniques to prevent payment fraud
Machine learning is a method of data analysis and automated model building. It is based on the idea that systems can learn from data and identify patterns with minimal human intervention. A TMS with machine learning capability can help uncover known and unknown cyberattack scenarios in real-time. Here’s how it works:
The TMS uses machine learning techniques to analyze the company’s financial data and detect payment anomalies and novel events. These outliers often indicate illegal activity such as fraud, identity theft, or network intrusion. There are many different approaches to identifying outliers in financial data.
Data plot
The simplest, but also the most manual way to detect irregularities is to plot the data in a two-dimensional space and then recognize patterns with the naked eye. This can be helpful when analyzing data for the first time. But it is neither a sustainable, efficient solution. Moreover, this method can only ever compare two sets of data.
Data clusters
A much better approach is to use machine learning tools to identify clusters in the data. Everything that cannot be assigned to a cluster is an outlier. The challenge with these methods lies in the choice of features to be investigated, and also in the chosen sensitivity of the algorithms.
Probabilistic approach
The statistical methods of machine learning are contrasted with probabilistic approaches such as the Bayesian estimator. Instead of recognizing patterns, the probability distribution of the data is approximated here. If a new value lies outside the variance, the probability of an outlier is very high.
First-digit law
Benford’s Law takes a completely different approach. It describes a regularity in the distribution of the leading digits of numbers in empirical data sets. Among other things, this property is applied worldwide in the verification of tax returns.
Time series analysis
One could also look at the problem from a different angle and instead of forming clusters, try to find irregularities in the time series of the data. By learning the time series with the help of machine learning techniques, the comparison of predictions with actual values can make possible outliers visible. A common model used is the Hidden Markov Model.
To prevent fraud, people and technology need to be aligned. People need to understand the security policies, and the threats and attack scenarios they should help prevent. Technology needs to be up-to-date and use the latest advances. Machine learning is best suited to complement standard anti-fraud measures. A TMS powered by machine learning can react in real-time to known and unknown attack scenarios by using information hidden in your financial data.
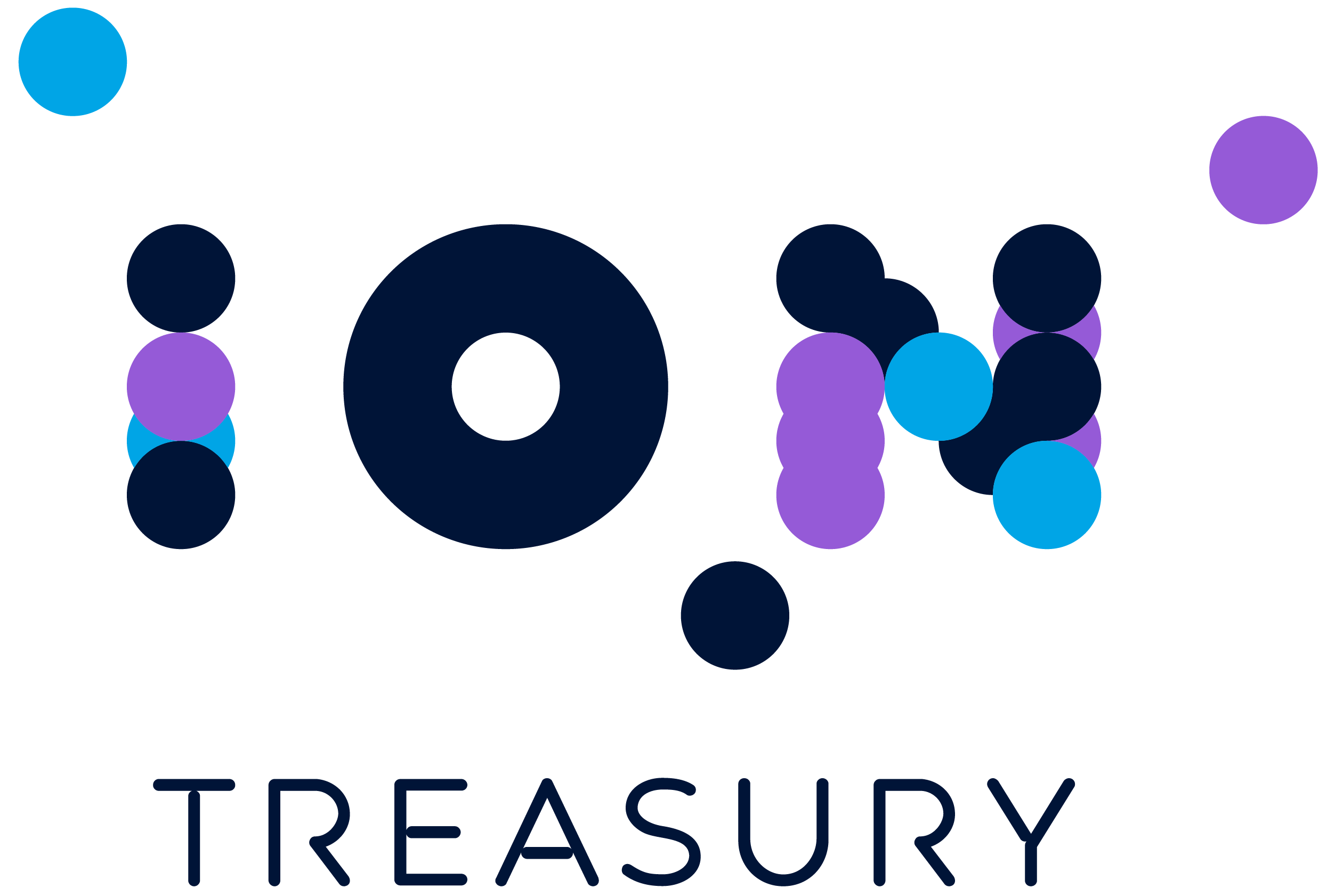
Machine learning by ION Treasury
If you want to learn more about the potential of artificial intelligence and machine learning in treasury, read our brochure.