How Machine Learning improves cash forecasting
COVID-19 has led to great uncertainty. Creating accurate cashflow forecasts is more difficult, particularly in the longer term. That’s why companies are making forecasts more frequently and with a shortened forecast window. Technology can help with these new requirements. Read this blog to learn about cash forecasting technology and how machine learning can help you navigate through the COVID-19 pandemic.
Requirements for cash forecasting
Cash forecasting is a challenging discipline. Depending on the organisation, processes, and tools, it takes days, weeks, or even months to put together the cash forecast. In times of uncertainty, speed matters more than ever. Companies need to be able to answer the following questions at any time:
- Do we have sufficient liquidity?
- Where is our cash and is it readily available?
- What are our sources of liquidity?
- What options exist to preserve our liquidity?
To answer these questions, treasury teams look at their cash position and cash forecasts, which give visibility across banks, accounts, and currencies, and the ability to forecast accurately and understand where funds are going. The cash position shows where your cash is and captures your actions for the day to help you accurately determine whether you need to invest or borrow. The cash forecast is a schedule of known payments, forecasted payments, and receipts. It helps organisations plan their liquidity out a week, month, quarter, or year.
Tools for cash forecasting
With spreadsheets, staff must manually log in to every bank account and any other portal from which they need information for their day’s work. While a degree of visibility is achievable using only Excel and bank portals, it proves labour intensive, error-prone, and slow.
As treasury teams are being asked to create more cash forecasts in a shorter period of time, their inefficient technology stack is holding them back even more. They start looking for automated tools to streamline their cashflow forecasting process. They are moving away from spreadsheets and bank portals onto treasury management systems (TMS). Treasury systems automate financial processes across the enterprise. They come along with best practices for cash, liquidity, and risk management and help companies increase efficiency, visibility, and control.
Innovative treasury systems use machine learning to simplify the cash forecasting process and reduce the time needed to produce a forecast. Using machine learning techniques, treasury teams can derive monetary gains, better prepare and plan, and validate data. Also, with these tools, they can be relieved from performing mundane, tedious tasks, allowing them to be a more strategic player and operate in new and more effective ways. Here is how machine learning can improve cash forecasting:
- Drastically reduces the time taken to produce forecasts: With machine learning techniques, cash forecasts can be generated 3,000 times faster than manually.
- Removes human biases and improves forecasting accuracy: Machine learning techniques such as neural networks can make cash forecasts by 10% more accurate.
- Realise monetary gains from more predictable cash requirements: For example, increasing the cash forecast accuracy by 10% on a 75 million USD average cash balance that’s invested at 2%, leads to a 150,000 USD gain per year.
Cash forecasting powered by machine learning in times of COVID-19
With large volumes of cash transaction data, originating from various sources, detecting historical trends and patterns can be impossible for the human eye. Machine learning can help treasury teams navigate through all this information to automate and improve cash forecasts.
Machine learning techniques depend very much on historical data and the trends and seasonality within the historical data. Events like COVID-19 create a lot of market volatility and many companies may view their historical data as “irrelevant” or “incorrect”. They wonder if they will be able to create reliable predictions on that data.
Indeed, the data used to train the machine learning algorithms ultimately affects what the generated forecast looks like. The better the quality of the input data used for training, the better the output will look. Still, machine learning techniques can help you improve the quality of your cash forecasts.
There is industry trend data and other statistics that are readily available on the market that you can apply to your data. In this scenario, the machine learning technique combined with scenario analysis capabilities will allow you to efficiently create your cashflow forecast and adjust it with a certain percentage change, so that it better matches your expectations of market impact. Using high-end technology will also allow you to create the forecast quickly and easily.
As machine learning and artificial intelligence developments in the chess world illustrate, treasurers will find that the right combination of efforts from machines and humans can yield major benefits for their departments and their organizations. Machine learning will reveal connections that lead to meaningful insights, and humans will use their company-specific knowledge to develop strategic workflows and influence key business decisions. In short, humans will still be needed to make decisions, but those decisions will be better informed and based on greater volumes of analysed data.
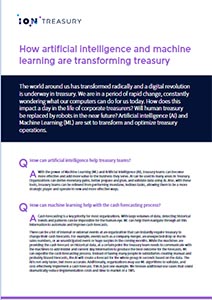
How Artificial Intelligence and Machine Learning are Transforming Treasury
If you want to learn more on the potential of artificial intelligence and machine learning in treasury, read my article.